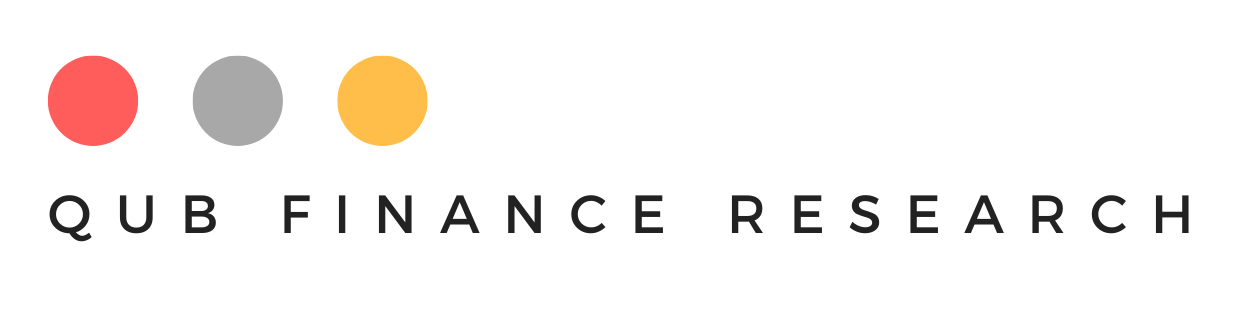
Background and Context
Research Focus
The study investigates how global common volatility (COVOL) and geopolitical risks affect clean energy prices using daily data from 2001-2024.
Market Context
Global investment in energy transition reached $1.8 trillion in 2023, with clean energy markets attracting diverse investments amid increasing volatility.
Methodology
Uses explainable artificial intelligence (XAI) methods including LASSO regression, Elastic Net, K-Nearest Neighbors, LightGBM, XGBoost and Extra Trees to analyze predictive power of risk factors.
COVOL Outperforms Traditional Models in Predicting Clean Energy Prices
- Extra Trees (ET) model achieves highest prediction accuracy with R-squared value of 0.9336
- Advanced machine learning models significantly outperform traditional statistical approaches
- LASSO and Elastic Net show poorest performance with R-squared values below 0.1
Model Performance Metrics Show Superior Results with COVOL
- Model incorporating COVOL shows lowest error rates across all metrics
- Mean Absolute Percentage Error (MAPE) of just 7.9% indicates high prediction accuracy
- Low RMSLE value of 0.1515 suggests consistent performance across different price ranges
Performance Improvement with COVOL vs Base Model
- Adding COVOL reduces prediction errors by up to 26%
- R-squared value improves by 2.57%
- Consistent improvement across all performance metrics
Comparative Performance During Ukraine Crisis Period
- Extra Trees maintains best performance during crisis period with lowest MAE of 7.1797
- Traditional models show significantly higher prediction errors
- Advanced machine learning models remain robust during market turbulence
Contribution and Implications
- First study to comprehensively examine how clean energy assets respond to COVOL and geopolitical concerns
- Demonstrates superiority of XAI framework in capturing complex market relationships
- Provides practical guidance for policymakers in creating risk management frameworks for clean energy markets
Data Sources
- Model comparison chart based on Table 4 showing performance metrics across different models
- Error metrics visualization derived from Table 2 performance statistics
- Improvement percentages from Table 7 showing model enhancement with COVOL
- Crisis period performance from Tables 17 and 18
- Feature importance visualization based on SHAP analysis results discussed in Section 4.2